
Reciprocal averaging
Graphics
- Developed in the 1930's; first introduced in ecology in 1973
(Hill, M.O., J. Ecol. 61:237-249)
- Synonymous w/ correspondence analysis, reciprocal ordering, dual
scaling
- Hill noted that a problem w/ PCA on variance/covariance
matrix is that information in rare species is not used in
the analysis. RA was proposed as a solution to this
problem; other advantages were noted later.
- Consider an environmental gradient:
- Assume that species B has the same mode as A (i.e.,
maximum expression is in same place along gradient),
but B is less abundant
- i.e., A and B have identical distributions, but if
you
put them in a V-C matrix,
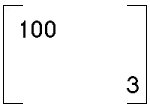
- and then do PCA on the V-C matrix, B (w/ small
variance) will be near the origin; A (w/ large
variance) will be far from the origin:
- i.e., A and B appear to have ecologically different
behaviors (distributions), but they don't! They have
identical modes
- PCA on the correlation matrix is more intuitively
palatable than PCA on the variance-covariance matrix in
this case
- RA will give a result similar to PCA on the correlation
matrix for this simple example
- An additional problem associated w/ PCA is called the "arch"
(or horseshoe) effect
- PCA forces data onto 2 (or more) axes, even if only one
gradient is influencing data
- RA reduces the "arch" problem compared to PCA [e.g., Fig.
4.14, Pielou 1984]
- Algorithm of RA involves simple matrix algebra on weighted
averages
- Weighted averages were used in DGA (ref. soil moisture
gradient of Dix and Smeins)
- RA scores converge to a unique solution which is independent
of initial arbitrary species scores
- RA tends to ordinate species together based on similar
distributions (regardless of abundances)
- i.e., species scores are adjusted for abundance
- Species w/ restricted distributions exert considerable
influence over ordination results
- These results may not be ecologically meaningful (i.e.,
outlying points may not be reflecting environment, but
sampling intensity)
- Therefore, species which occur infrequently
(arbitrarily, > 5% of quadrats) are often removed
from
data set before analysis
- All species and quadrats in any ordination influence
the final ordination
- Both PCA and RA are linear models
- i.e., they assume species are distributed in a linear manner
- Projections of non-linear data onto fewer axes than found in
the original data gives a misleading picture of the original
data (and probably misinterpretation) [ref. Fig. 4.12 Pielou
1984, p. 189]
- Departures from linearity in real data are expressed by
[ref. Fig. 3.15 Gauch 1982, p. 106; Fig. 4.14 Pielou
1984, p. 193]:
- "arch" effect
- involution of secondary axes
- scale contraction at the ends
- These problems are bad enough w/ simulated data (when we know
what the gradient is)
- With real data, gradients influencing vegetation
probably are unknown--that's why we're doing ordination
- Note that RA is more robust than PCA to nonlinear relationships
between species performances and environment ("arch" effect,
involution, and scale contraction are all less pronounced)
- A further "advantage" of RA over PCA is the ability to include
environmental data in the ordination, according to Gauch and
Stone (1979, Amer. Midl. Nat. 102:332-345; cited in Gauch 1982)
- Environmental interpretation follows ordination
w/ PCA, but can be included w/ RA
- Environmental variables are treated as "species" for
each quadrat, and numerical values of environmental
variables are analogous to species abundances
- With any ordination algorithm, correlation coefficients can
be calculated between environmental variables and ordination
axes
Detrended correspondence analysis
More
graphics
- Detrended correspondence analysis (i.e., detrended reciprocal
averaging)
- developed in software form (DECORANA) in 1979
- published in a journal in 1980 (Hill & Gauch Vegetatio
42:47-58)
- Goals are to remove "arch" effect and compression of first-axis
ends
- Method:
- To get rid of "arch", divide axis 1 into several segments,
and adjust axis 2 to have a mean of 0 in each segment
- To calculate a third DCA axis, sample scores are detrended
w/ respect to the second axis as well as the first ... and
so on for higher axes
- Several authors (e.g., Pielou, ter Braak) have suggested
that DCA is "overzealous" in its removal of the "arch"
- A second method for eliminating the "arch" was suggested
in
1987 (ter Braak, C.F. Vegetatio 69:69-77)
- "Arch" reflects quadratic relationship between first
axis and second axis
- In addition to the constraint that axes be orthogonal,
merely add a second constraint: that axes be
uncorrelated w/ the square (cube, etc.) of previous
axes
- termed "detrending by polynomials", done w/ ter
Braak's (1987) canonical correspondence analysis
computer program, CANOCO
- The second goal of DCA is to "stretch" axis ends
- this causes distances in the ordination space to have
consistent meaning in terms of compositional
differences of samples, or distributional differences
of species
- DCA is currently popular w/ ecologists, esp. w/ nonlinear data
- The most common criticism of DCA is that detrending is
artificial
- problems ("arch", compression of axes) are "fixed" whether
they are real or not
- w/ field data, we don't know relationships between species
and quadrats
- Interpretation and presentation of ordination results (common
display options):
- Quadrat or species lists
- rank order of ordination scores may present a clear
gradient (e.g., moisture, successional status)
- Arranged matrix
- not very useful if
2
gradients (still appears random)
- Quadrat and species ordination graphs
- if
2 axes,
use a series of graphs (e.g., plot 1*2, 1*3)
- Graph environmental parameters on quadrat ordination
- instead of plotting points for each quadrat, plot value
of some environmental variable (e.g., pH); isolines are
sometimes drawn
- Hybrid ordination
- allows various kinds of data to be plotted on one
figure
- however, since each "species" (incl. environmental
data) and quadrat affects the ordination, it may be
undesirable to include environmental data in ordination
- Trace diagram
- ordination score for one axis (usu. axis 1) and
environmental data, plotted by plot location
Previous
lectureNext
lecture